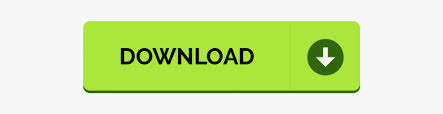
■ Note: this is the residual variation if the null model is used, so there is no further decomposition possible for that model. ■ Total variation is assessed by squaring the deviations of the responses around the overall or grand mean (γ̿, the estimated mean for all the observations and available from the mean-only model). ■ By summing over all nj observations in each group and then adding those results up across the groups, we accumulate the variation across all N observations. We define each of these quantities in the One-Way ANOVA situation as follows: With multiple groups, we will focus on decomposing that total variability ( Total Sums of Squares) into variability among the means (we'll call this Explanatory Variable A's Sums of Squares) and variability in the residuals or errors ( Error Sums of Squares). It is developed using sums of squares which are measures of total variation like used in the numerator of the standard deviation that took all the observations, subtracted the mean, squared the differences, and then added up the results over all the observations to generate a measure of total variability. The statistic is called the ANOVA F-statistic. So we need a test statistic to help us make some sort of comparison of the groups and to account for the amount of variability present around the means. In the plots, there are two sources of variability in the responses - how much the group means vary across the groups and how much variability there is around the means in each group. Consider alternate versions of each result in Situations 3 and 4 and how much evidence there appears to be for same sizes of differences in the means. Why? It is because the variation in the means looks "clear" relative to the variation around the means. In Situation 1, it looks like there is little evidence for a difference in the means and in Situation 2, it looks fairly clear that there is a difference in the group means. Figure 2-3: Demonstration of different amount of difference in means relative to variability.Ĭonsider the four different fake results for a situation with four groups in Figure 2-3. It is basically what is left over that the mean part of the model (μ̂ j) does not explain and is our window into how "good" the model might be. An estimated residual (e ij) is the difference between an observation, γ ij, and the model estimate, γ̂ ij=μ̂ j, for that observation, γ ij - γ̂ ij=e ij.
The sample size in each group is denoted n j and the total sample size is N=Σn j = n 1+n 2+.+n J where Σ (capital sigma) means "add up over whatever follows". In order to develop the test, some additional notation needs to be defined. In this section, we develop what is called the ANOVA F-test that provides a method of aggregating the differences among the means of 2 or more groups and testing our null hypothesis of no difference in the means vs the alternative.
ASREML MEAN SQUARE ERROR R HOW TO
The previous discussion showed two ways of estimating the model but still hasn't addressed how to assess evidence related to whether the observed differences in the means among the groups is "real".